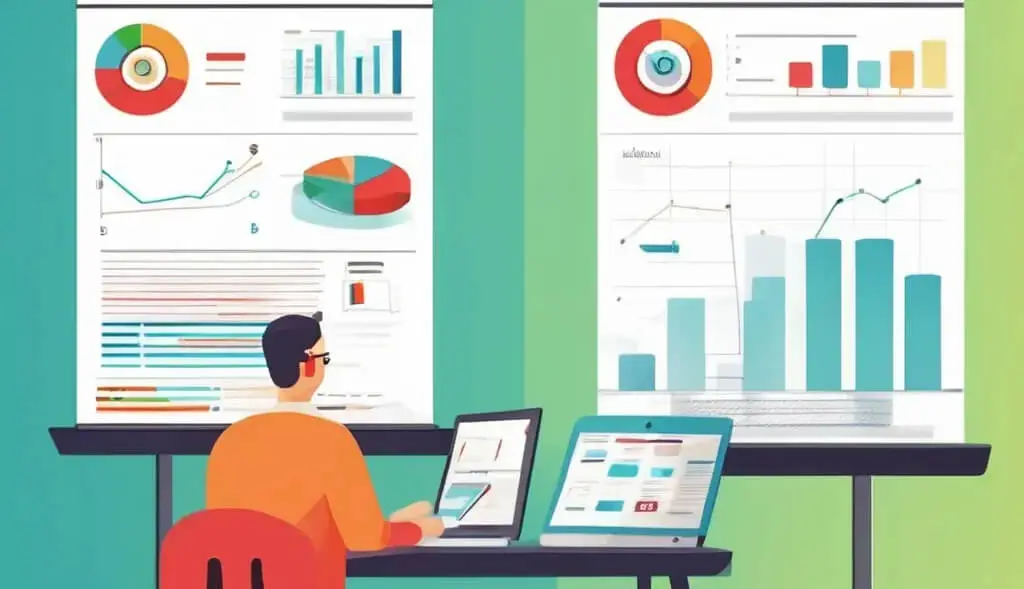
When you develop a new product or change the design of a product, would you like to know in advance what the impact will be? A/B testing unravels the mystery behind this process, allowing us to compare the performance of two different options. Used in a wide range of fields from digital marketing to financial services, this method is indispensable for analysing users' preferences. Let's explore A/B testing and discover ways to increase our success with data-driven strategies together!
A/B testing is a statistical method that allows us to choose the more advantageous one by analysing two strategies. With A/B testing, we can find the advantageous one by comparing different advertising strategies, designs and products. So how is A/B testing done? Let's continue!
In order to implement A/B testing, the target, evaluation metrics and variable should be determined first. For example, we may want to sell to more customers by changing the colour of a product. A/B testing is used to determine whether we can sell more when we change the colour.
The necessary steps to start the A/B test have been completed. So what then? This stage is the data collection stage. Which product customers or users prefer is observed at this stage. Users are divided into two groups. The first group (Control Group) experiences scenario A, while the other group experiences the new product. The data collection phase continues for a certain period of time.
At this stage, the results obtained in the AB test are evaluated. The performance of the two scenarios is analysed statistically. For example, one scenario has 5 percent more sales than the other. Is this rate statistically sufficient? Does this result meet the expectation? answers are searched for.
After the results are evaluated, a decision is made for the AB test. If the results are not statistically significant enough, the AB test is continued. If the AB test is significant enough, the performance of the scenarios is evaluated. If the new product performance does not meet the expectations, the old product continues to be used. If the new product performs as expected, this product is started to be used and marketed.
The A/B test method is an important technique that is actively used in many sectors. We can examine the important areas where A/B testing is used below.
• Digital Marketing
• Web and Application Development
• Product Development
• Advertising Strategies
• Sales and E commerce
• Financial Services
A/B testing is a powerful statistical method used in marketing to compare two versions of a campaign or item to determine which one performs better. With A/B testing, which is becoming increasingly important especially in the digital marketing sector, it can be analysed which product will be offered to which users. By presenting scenario A to one group and scenario B to another, marketers can analyse the impact of different variables such as headlines, visuals, designs, campaigns or calls to action on customer engagement and conversion rates. This data-driven approach allows for more informed decisions, optimising campaigns for better results and maximising ROI. With these advantages, A/B testing is an important tool for improving marketing strategies based on real user behaviour.
• Google Optimize: It works integrated with Google Analytics to analyse user behaviour and perform tests.
• A/B Smartly:
• AB Tasty: Provides tools to improve the user experience, designed specifically for marketing teams
• Optimizely: It is an advanced A/B testing platform known for its user-friendly interface and powerful features and is suitable for large-scale projects.
• VWO (Visual Website Optimizer): It offers comprehensive tools to analyse user behaviour and increase conversions.
• Adobe Target: It is an A/B testing platform that stands out with its advanced targeting and personalisation features.
• Hotjar: It is an advanced A/B testing platform that offers qualitative analysis tools such as heat maps and session logs to understand user behaviour.
• Crazy Egg: It is an advanced A/B testing platform that analyses user behaviour with heat maps, scroll maps and user logs.
• Convert: It is a user-friendly A/B testing tool that is especially suitable for small and medium-sized businesses.
• Kameleoon: It is an innovative A/B testing platform that offers AI-powered personalisation and A/B tests.
• Unbounce: It is an A/B testing platform that enables A/B tests for landing pages and focuses on increasing conversion rates (CVR).
• SplitMetrics: It is a platform for conducting A/B tests for mobile applications and games.
• LaunchDarkly: It is a platform that offers A/B tests especially for software development teams.
So why should we do A/B testing, why is A/B testing important? A/B testing is an important method to make decision-making processes data-driven. With A/B testing, the decision-making process becomes more scientific and reliable. Potential risks are reduced with the use of this method. Success rate and customer satisfaction increase in strategies created using AB testing. This is a very important advantage for both individuals and brands.
Split A/B testing is a method for measuring the effect of a single variable on performance. With the Split AB test, two different versions of a product or design (A and B) can be compared on a single variable to determine the better version.
In this AB test method, combinations of multiple variables are analysed. For example, suppose we want to change the colour and size of a button on an interface. In this case, we can analyse the colour and size variables together. We can do this analysis easily with Multivariate test.
In order to interpret the results of the A / B test, we can examine certain performance metrics and make decisions according to these metrics. Since A/B testing can be used in every sector and project, success metrics will vary according to these projects. To examine the button example, we can use the click rate of users as a metric in this analysis. We can also make our decision according to the change in the click rate.
The important point to be considered when analysing performance metrics is that the results should be statistically significant and reliable. The results obtained should be statistically evaluated by establishing a hypothesis test and examining the confidence level. As a result of this evaluation, if the AB test is significant enough, the scenario with better performance is considered successful. If there is not enough statistical confidence, the AB test is continued.
If you want to be informed about the developments and case studies about statistics, you can follow me from the accounts below.
Linkedin: linkedin.com/in/mustafabayhan/
Medium : medium.com/@bayhanmustafa